Just when you thought you had mastered Generative AI, large language models, and the idea that AI can be conversational, the next evolution of AI is already here. There’s a new buzzword on the scene. It’s called “Agentic AI.” But while Gartner analysts and tech futurists are touting this technology as the new multi-trillion-dollar opportunity that will change everything, no one really knows what Agentic AI is. Moreover, everyone is defining it a bit differently.
As Billtrust’s SVP of AI, I am here to help bring some clarity to this term, drawing some clear lines between reality and hype. I’ll also explain Billtrust’s approach to Agentic AI and how our strategy differs from others in the accounts receivable automation industry. Let’s start with the basics.
What is Agentic AI?
Agentic AI is the next generation of Generative AI and refers to AI-powered virtual assistants or “agents” that can act autonomously. Agentic agents use GenAI technology and other tools which enable reasoning capabilities and the pursuit of goals. By reasoning through multi-step processes, these agents can execute complex workflows in ways that simply weren’t possible with predecessor technologies. Most notably, they can act independently, without human intervention. For instance, Agentic AI goes a step further than traditional Q&A chatbots, virtual assistants, and even GenAI content generators -- empowering its intelligent agents to take full ownership of tasks, collaborate with other systems, and even adapt to changing conditions.
The impact is huge: Agentic AI is giving professionals across every industry their own hyper-intelligent agents that can act alone, making complex data-driven decisions and carrying out appropriate responses to situations.
Different definitions and capabilities: Not all agents are the same
There’s a lot of variation in the definition of “agents.” Even the tech heavy weights like Amazon and Google differ in their descriptions. To further muddy the waters, Microsoft tends to differentiate between agents and assistants, while others don’t.
Definitions aside, it’s more about what Agentic agents can and cannot do.
Capabilities come with a significant amount of variability. This is largely because Agentic AI, although available today, remains an emerging toolset for most providers. As with any new technology, maturity will vary widely and buyers should be prepared for marketing hype, potential false claims, and a broad spectrum of actual abilities.
Cutting through confusion: Automated workflows versus autonomous agents
False claims create a real headache for corporate buyers but Anthropic offers tangible clarity. They recognize that Agentic systems may cover everything from predefined workflows to fully autonomous AI models, but they draw an important distinction between workflows and agents:
- Workflows: “systems where large language models and tools are orchestrated through predefined code paths”
- Autonomous Agents: “systems where LLMs dynamically direct their own processes and tool usage, maintaining control over how they accomplish tasks”
Action-based agents are the key dividing line. Providers may claim a high number of agents, but that count may amount to nothing if the provider simply labels their automated workflows “autonomous Agentic agents.”
Buyer Beware
Just because 100% of a task is automated doesn’t necessarily mean that work is performed by a fully autonomous agent. Providers may sell their software platforms based on a large volume of Agentic AI agents, but that volume may not equate to a larger “volume of value.” When built correctly, a single agent can do many tasks, and a handful of expertly crafted agents can perform thousands of tasks without prompting. Buyers should be prepared for marketing hype, potential false claims, and a broad spectrum of actual capabilities.
Now that we have defined what an actual Agent is, we can address its impact based on different levels of sophistication – that is – it’s ability to combine the power of large language models, reasoning capabilities, and contextual financial data into a high-value autonomous assistant for AR professionals.
Parsing the difference between automated workflows and Agentic agents
When in doubt, buyers should inquire about how agents were built and trained, defining specifically when and how the human is brought back into the process. You might ask: “Does this agent follow prescriptive or predefined workflows and identify anomalies where the human needs to intervene?” “Can it really operate autonomously for extended periods of time?” Or even, “Tell me more about how your Agentic agents are trained and the data used to train them.” (See the “Data engineering matters” section below for more on this.)
The clearest picture of Agentic AI: the culmination of tools
To get the clearest view of Agentic AI’s transformative power, it helps to paint the full picture by showing you everything that gets folded in. For example, the following capabilities may be considered elements of an Agentic AI system that converge to deliver the heightened value of full autonomy:
- Automated workflows that get smarter with time
- Conversational chatbots or virtual assistants
- AI-generated predictions and recommendations that solve problems proactively
- Autonomous AI assistants that can act on their own recommendations
Agentic AI is groundbreaking because it unites all of today’s tools while enabling multiple autonomous agents to work collaboratively across software systems and large ecosystems. You can see how it builds on GenAI, developing the next evolution of AI.
Here’s a tangible example that contextualizes how multi-agent Agentic AI takes GenAI one giant leap forward. GenAI can tell you how many flights there are going from your city to Paris. But Agentic AI can see an email from your boss asking you to book a flight to Paris and book it based on predicted weather conditions and data showing which flights are most likely to arrive on time. This shift demonstrates how next-gen agents advance the utility of AI in business processes.
What does Agentic AI mean for accounts receivable?
In the field of AR, Agentic AI is a game changer. That’s because much of the work today is siloed, manual, and highly repetitive – ripe for high levels of autonomy. Here’s a quick look at some of the use cases for Agentic AI in AR.
Agentic AI use cases across the AR lifecycle | |
---|---|
Invoicing |
Physical address databases that autonomously maintain their accuracy Predictive personalization engines can dynamically redesign invoice formats to improve payment completion, editing due date placement, payment button colors, layout, etc. Agents enable global compliance monitoring by maintaining always-on regulatory alignment |
Payments |
Automated payment policy calibration that dynamically responds to buyer behaviors Self-optimizing payment processes that continually minimize delays Agents infer payments occurring outside the Billtrust software to offer insights across the full spectrum of payments activity Read and extract ACH remit data sent from ACH providers |
Cash Application |
Autonomous reconciliation processes ensure humans only work on exceptions Payor monitoring recognizes when influential payors unexpectedly downgrade in matching ML-powered data extraction and matching maximize straight-through processing Proprietary, confidence-based algorithms intelligently adapt and deliver incremental match rate increases, eliminating errors and driving accuracy Data reconstruction capabilities autonomously piece together fragmented remittance data using historical patterns and cross-system intelligence to reduce unapplied cash and accelerate liquidity |
Credit & Collections |
Personalized collections communications at scale for improved buyer experiences Dynamic credit lines that identify and mitigate risk while maximizing new sales opportunities Autonomous dispute resolution Collections agents boost staff efficiency by expediting email response and intelligently prioritizing queues Credit monitoring that goes beyond real-time monitoring to predict risk |
Holistic Management and Oversight | |
|
Get the complete eBook “From GenAI to Agentic AI: Accelerating Cash Flow Management with Artificial Intelligence” to learn more about how Agentic is transforming AR.
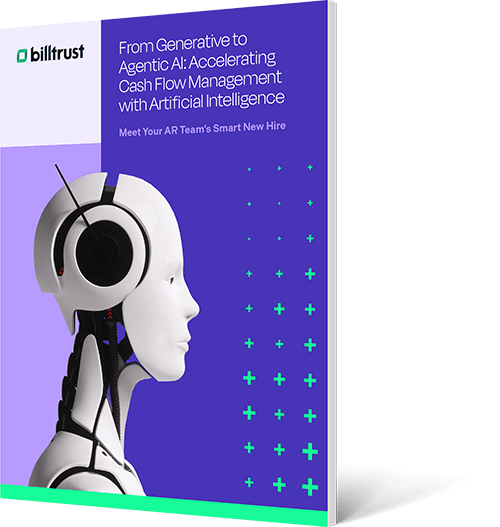
Cash application reconciliations, for example, are low hanging fruit for total transformation. Comparing invoice data to payment data is a task set that has already been automated thanks in part to machine learning. Now, AR software providers are further building on this groundwork, advancing traditional AI automation into autonomous agents.
Buyer Beware
Be wary of AR platforms already claiming autonomous agents for AR cash application processes, as these can be what Anthropic describes as automated workflows rather than true autonomy. For instance, integrations and robotic process automation aren’t agents. Sophisticated AR agents are marked by an understructure of large language models, reasoning capabilities, other tools, and financial data.
With time and maturity, financial teams will expand Agentic AI across the AR ecosystem, with agents increasingly collaborating across multiple software systems. This will transform the way B2B payments are handled. Agents will communicate between entities, such as banks and buyers, mimicking human interactions. As this happens AI will be embedded into the world of payments. Ultimately, there will be a shift towards task-based payment models, where companies pay for specific tasks completed by AI agents rather than traditional subscription models.
The impact on AR professionals
The rapid advancement of Agentic AI models requires businesses to continuously update their technical and business skills to effectively adopt and utilize these models. While AI significantly augments today’s teams, it is not yet removing the human entirely from AR work. AI can recommend AR strategies, but human expertise is still crucial for crafting these strategies.
This is why AI literacy is critical for AR professionals at all levels.
Roles are transforming as AI elevates employees into new responsibilities. Instead of data managers, we’re all becoming AI trainers and creators of AI-driven predictions that will help uncover new financial opportunities. Future AR knowledge workers must be able to articulate business strategy and build their personal experience into Agentic workflows. AI benefits reach beyond simple time savings. It’s creating a chance for professionals to co-create the next generation of financial systems. Professionals that ignore this opportunity will be more vulnerable to AI job displacements. Embracing Agentic agents enhances the resume.
The Real Job Risk
The true job risk isn't just AI itself, but the advantage gained by individuals who utilize Agentic AI to surpass the performance capabilities of those who don't or haven't had the chance to use it.
Understanding the Agentic AI market and how Billtrust stands apart
With Gartner calling Agentic AI “the next big thing,” the competitive landscape for AR automation software is a hot bed of activity. New entrants are AI-first startups, and they’re challenging incumbents by advertising themselves as “more modern solutions.” They label +20-year pioneers like Billtrust as “traditional approaches” lacking the newer AI technologies of startups.
Of course, nothing could be further from the truth.
The cost of AI has significantly decreased, making it more accessible to all software providers -- large and small, experienced and inexperienced. In fact, Billtrust takes a modern, GenAI-first approach to advancing our AI automation.
Just like startups, Billtrust takes a GenAI-first approach to AI innovation.
What does a GenAI-first approach mean?
A GenAI-first strategy is considered the “next-generation approach” or “more modern” approach to AI innovation. It’s the strategy that AI-startups claim. Traditionally, AI automation has started with machine learning and advanced AI analytics. While these tools are still tried-and-true strategies for automation, companies can achieve robotic process automation in different ways today -- most notably, starting with large language models.
Billtrust uses this Gen-AI first approach. In fact, our Co-Pilot AI platform is powered by LLMs, and we continue to advance it into action-based Agentic agents.
Now that we’re all equals here. Let’s talk about differences.
The difference between AI-startups and Billtrust is in the data
Startups take data from a very small, immature book of business and apply AI tools to it.
That’s a very different story when compared to Billtrust.
Thanks to our 20 years in the business, we have the industry's largest financial data network. We take 20+ years of anonymized data from across the AR lifecycle and train our AI platform on it.
Data engineering matters
We all know that AI models are only as smart as the data they’re provided. Data engineering matters. Ingesting enterprise-level data at scale should be considered a prerequisite for any AI system. After all, vast datasets facilitate common data models which give Agentic agents the ability to “know” how to act and respond to different scenarios. Data is a big deal, because it determines how the agent will generate recommendations or personalized experiences for example. AR Providers that don’t have enterprise data engineering capabilities tend to gloss over this foundational necessity.
Billtrust integrates enterprise data at scale, including $1T in synthetic transactional data annually. Very rarely is there a situation or scenario that our AI system hasn’t seen before. This means our AI platform can outsmart our competitors, offering clients unparalleled visibility and control, more accurate industry benchmarks, and autonomous capabilities derived from more than two decades of hands-on AR experience.
AI is just a tool. Without a wealth of quality data and the right industry experts guiding it, AI cannot deliver powerful insights and transformative business outcomes.
Here’s what else you should know:
- Startups often start with point solutions specialized in specific areas of AR, meaning they can provide interesting but disconnected insights or isolated recommendations and actions. However, Billtrust’s solution covers the gamut, so you get a coordinated, multi-agent response team across your entire AR ecosystem.
- Startups may address a small number of client transactions, but Billtrust handles $1 trillion payments annually. We understand transactions and all the nuances that come with them -- from credit card processing fees to the industry’s business payment networks.
- Startups and point solutions may not integrate with all your financial systems and tools. Meanwhile, Billtrust provides 40 ERP connectors and invoices 260 AP portals regularly, meaning our AI collaborates across your AR ecosystem providing coordinated intelligence and embedding deep domain experience into every recommendation and autonomous agent.
- Startups have limited AR feature sets that often lack the flexibility financial teams need to mold automation capabilities around their unique processes and business needs. Meanwhile, Billtrust offers some of the most customizable payment policies on the market today.
At the end of the day, decision makers should also ask themselves this key question: When you’re accelerating your cash flow and protecting your financial flexibility, would you prefer a startup that may or may not be around in 2 to 5 years, or would you rather bank on the security and safety of an industry mainstay with a proven AI track record?
Start your AI journey with Billtrust. Talk to one of our AI experts today for a free consultation.