“Imagine a team of virtual experts tailored to your business working tirelessly behind the scenes to optimize your entire AR process. It’s something that could be here sooner than later,” says Ahsan Shah, SVP, AI & Analytics, responsible for driving the AI evolution at Billtrust. He believes in the transformative potential of multi-agent AI, a cutting-edge approach that goes beyond traditional AI by employing multiple intelligent agents, each specialized in distinct tasks, to collaborate towards shared objectives.
This article explores how multi-agent AI systems will revolutionize AR by optimizing the AR lifecycle and enhancing strategic decision-making. Ahsan Shah offers an insider's perspective and forecasts future trends and innovations poised to transform B2B SaaS accounts receivable software from 2025 onward.
Understanding AI agents
Before we explore the magic of multi-agent systems, let's clarify what an AI agent is. An AI agent is a sophisticated software program designed to act autonomously, making decisions and performing actions to achieve a specific goal. These agents can interact with their environment, learn from data, and adapt to changing circumstances. Think of them as highly intelligent assistants capable of handling complex tasks without constant human intervention.
Read the blog → Transforming accounts receivable with AI: Meet Billtrust’s Finance Co-Pilot
Introduction to multi-agent AI
Unlike single-agent systems, where one AI handles all tasks, multi-agent systems distribute tasks among multiple, specialized agents, each with its own specific task and expertise. Together, they form a cohesive system adept at tackling intricate challenges. By interacting with each other and their environment, agents collaborate to solve complex problems more efficiently than a single agent could alone.
This collaborative approach boosts efficiency and effectiveness, proving especially beneficial in managing the complex processes of AR. Like human organizations — such as your own finance team — multi-agent systems collaborate to achieve shared goals.
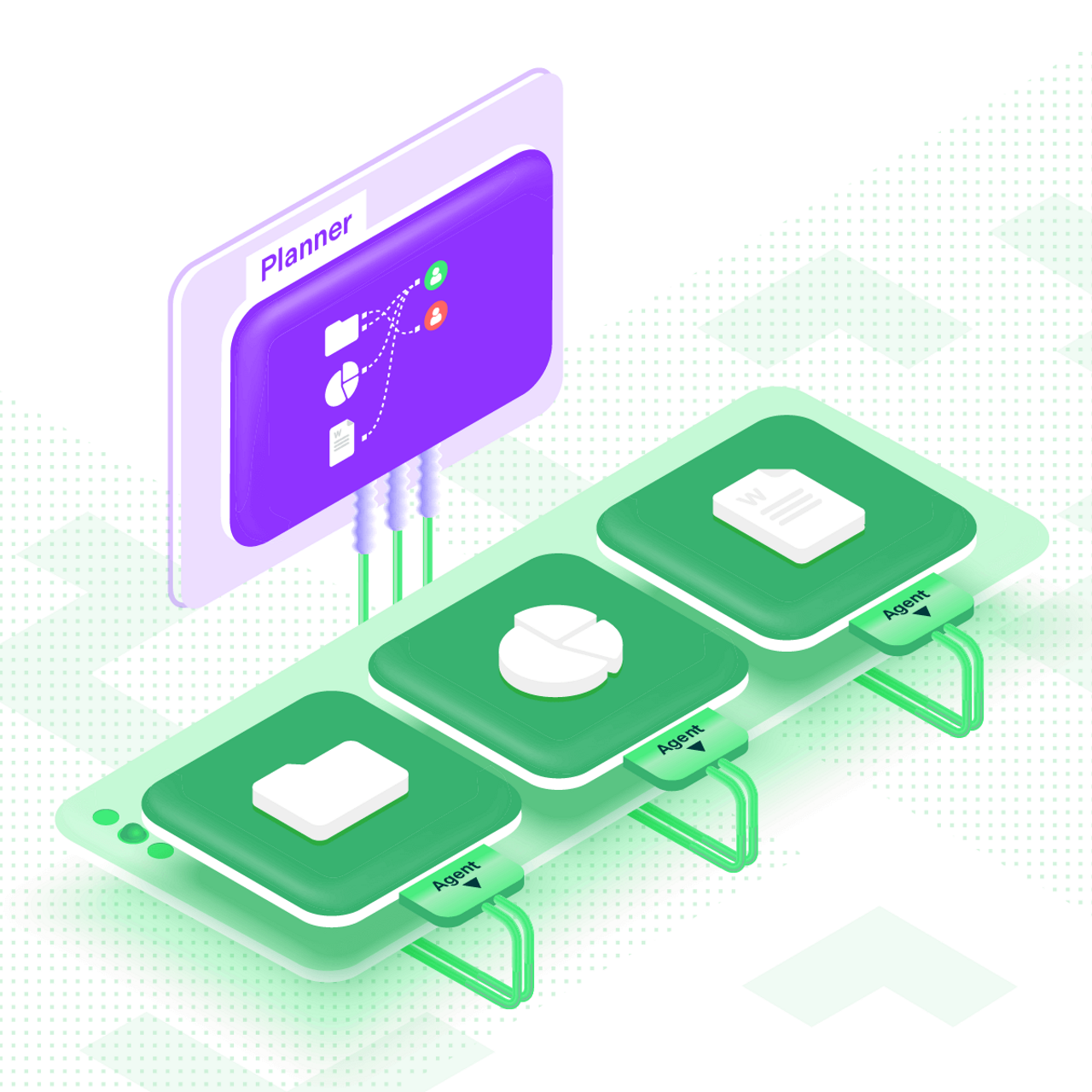
How specialized AR agents work together to optimize the AR lifecycle
"These systems will revolutionize various business processes, including accounts receivable," says Ahsan Shah. Specialized AR agents are AI entities designed to manage specific aspects of the AR lifecycle, such as collections, credit management, payments, and invoicing. Each agent is equipped with domain-specific knowledge and capabilities, enabling it to perform its tasks with high precision and efficiency.
Multi-agent AI systems are most powerful when specialized agents collaborate. These agents communicate and share information, allowing them to coordinate their efforts and optimize the AR lifecycle. For example, a collections agent might work with a credit agent to identify high-risk accounts and develop strategies to mitigate potential losses.
This type of AI goes beyond simple question-and-answer interactions. "Instead, agents can reason, plan, and execute tasks autonomously, like how a team of experts would work together in a company" says Ahsan Shah. "Essentially, we're teaching AI to work collaboratively. By giving it tools, support, and context, and setting objectives, it can reason and plan using a chain of thought."
AI as an advisor
Ahsan Shah: “This represents a substantial leap for AI in B2B AR software. What we’re seeing is the transformation from an AI agent, with a big human presence with premeditated questions, to AI as an advisor.”
“It enables AR professionals across all levels to focus on strategic goals, with multi-agent AI offering valuable insights, guidance, and working as a collaborative partner in the future finance organization. This will enable AR teams to significantly reduce human capital and allow for more sophisticated analyses.”
“At Billtrust, we’re currently creating this set of graphs with specialized agents, which will be given various tools, data access layers, and domain specific knowledge to enable organizational collaboration between Al artifacts. We’re starting with payments now, but are extending it across AR. Other scenarios could be a collections procedure optimizer or real time AI search.”
Ahsan Shah, SVP, AI & Analytics, Billtrust
Continuous improvement
What’s unique to this multi-agent approach is that this intricate network works dynamically and cyclically, continuously passing information and insights back and forth. The system runs perpetually in the background, like a dedicated team that allows your actual team to focus on more strategic growth objectives.
This optimization ensures that AR processes remain efficient and effective, adapting seamlessly to changing conditions and new information. “What’s great about it is that it can be hyper personalized to the specific needs of customers, industries and buyer behavior patterns,” says Shah.
The human element
The setup mirrors the current structure of an AR organization, much like a collections or credit manager interacting with payment policies, or a terms manager assessing credit risk.
This human element is important, articulates Shah. “The final action can be configured so that human workers can simply approve to apply the recommended configurations. Our philosophy on AI, particularly Gen AI, views it as a creative augmentation, rather than a replacement for human involvement.”
“What excites us about this ability to use agents, nodes, and graphs is that it allows us to take a higher-level business objective and have a system execute the objective in a non-deterministic way.”
Ahsan Shah, SVP, AI & Analytics, Billtrust
Read the industry report → AI pushing the boundaries of what’s possible for OTC
Translating business objectives into a plan
Multi-agent AI empowers you to go beyond simple questions, supporting you in achieving advanced business goals. As a supplier, navigating a vast array of buyers can be daunting, especially when it comes to analyzing data sets and personalizing buyer behavior.
Imagine wanting to identify the most relevant opportunities, assess risks, and provide recommendations based on the past three months of payment activity among your buyers. “For starters, this is a unique question,” says Shah, “since we haven’t defined what risk or opportunity means, nor do we know what a recommendation could be. And this isn’t just a question —it’s more of a higher-level strategic business objective.”
“With the payment policy optimizer we’re currently testing at Billtrust, we’re leveraging a graph to identify both opportunities, such as surcharging and payment grace periods, and risks, like low-margin customers and late payment penalties.”
The multi-agent AI system gathers initial data sets, examines buyer information, analyzes trends, detects anomalies, and identifies opportunities. It scrutinizes payment methods, terms, and Days-to-Pay for each buyer. By consolidating this information, it can reveal specific buyers who might benefit from switching to ACH.
Additionally, it can categorize higher-risk buyers where penalties may be necessary, providing suppliers with a hedge against low-margin transactions. Conversely, there are top-performing buyers—those who consistently pay well within their terms. These are opportunities to enhance relationships by offering them better terms, extending credit, or providing early payment discounts as a reward for their reliability.
A noteworthy feature is its ability to generate visualizations that can be easily integrated into PowerPoint or other presentations, thereby accelerating decision-making and action for customers.
The AI agent proceeds by presenting an executive summary, whether in an email or a document uploaded to the application. This summary encapsulates key findings, sources, outliers, early payers, risks, and recommendations. It delivers a tactical overview that results from thorough research across accounts receivable.
Ahsan Shah states, "The design of this interface within your software is still under discussion. However, it certainly won't resemble the Q&A interface you're familiar with in Finance Co-Pilot. We envision it as a back-end process operating systematically. Imagine arriving in the morning to a list of tasks for optimizing your payment policy, all generated while you sleep. We are deliberating whether this should be presented in-app or sent via email."
The Billtrust advantage: A leader in AI-powered AR
Billtrust is at the forefront of this revolution, investing heavily in AI-driven solutions to empower finance teams. "We don't look at AI as a silo; we see it as an integral part of our overall data analytics strategy, augmented by AI," Shah explains. Billtrust's phased approach to AI development ensures that we are continuously pushing the boundaries of what's possible in AR.
Ahsan Shah believes that using multi-agent tools in graph execution represents the future of B2B SaaS for enterprises. This vision for the future of B2B software extends beyond mere cost reduction. "It's about creating value and identifying new opportunities," Shah emphasizes. By empowering finance organizations to define their objectives, and then unleashing a team of AI agents to strategize and execute, Billtrust is paving the way for unprecedented levels of AR optimization.
Revolutionize your AR strategy with AI
Learn how AI can provide smarter insights and improve your AR lifecycle.
Learn more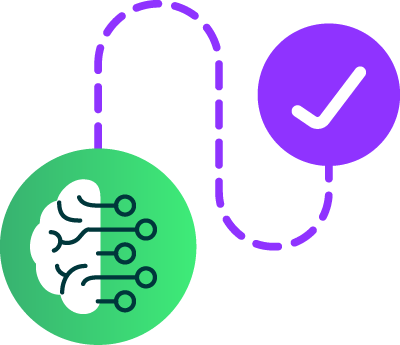
Embracing the AI revolution
Multi-agent AI presents an exciting opportunity for finance leaders to transform their AR processes and optimize the AR lifecycle. By adopting this cutting-edge technology, you can unlock unprecedented efficiency, insight, and strategic advantage. Leveraging the collective intelligence of specialized agents enables businesses to achieve continuous optimization and make more informed decisions.
Multi-agent AI has the potential to revolutionize not just accounts receivable, but the entire B2B software landscape. By enabling AI systems to function more autonomously and collaboratively, companies can unlock new levels of efficiency, insight, and value creation. As Shah notes, "It’s in early innings still, but we’re moving at a rapid pace."
As we move towards 2025 and beyond, the adoption of multi-agent AI systems is expected to increase, driven by advancements in AI technology and the growing need for efficient and effective AR management. Businesses that embrace these systems will be well-positioned to stay competitive and achieve their strategic goals.
Billtrust is committed to leading this charge, empowering businesses to navigate the future of finance with confidence and achieve unprecedented success.
FAQ
Now, how does such a graph look, so you’ll better understand what’s happening behind the scenes:
The outer graph controls the planning and workflow, while the inner graph (green) consists of the Agent, with a powerful combination of tools. The Planner and Agent interact in a constant and cyclical way and provide content to a Refiner node to summarize and recommend.
In essence, you have multiple AI artifacts performing specific functions to answer higher-order questions, according to this logical model or workflow:
- Planner: This strategic mastermind takes this question and dissects it into a series of manageable tasks for the AI Agent to execute. It's the conductor of the AI orchestra, ensuring every agent plays its part harmoniously. Ahsan Shah: “The planner has a clear objective: identify opportunities and risks and how to basically improve the business. The planner thinks about how it would achieve that objective. It will instruct the agent to execute a query, retrieve a set of data, look at different patterns, and then it’s finally going to go to the Refiner.”
- Human Interrupt: Allows you to continue with the plan or specify which parts of the plan you want to modify.
- Agent: The agent is a powerful node consisting of several tools: Supervisor, Researcher, Analyst, (Data) Visualizer, SQL Generator, and Coder.
- Replan: This element assesses our progress according to the original plan. Do we possess the necessary information or not?
- Refiner: This meticulous editor takes all the findings from the various tools and compiles them into a clear, concise report. It might generate an executive summary outlining key findings, risks, opportunities, and actionable recommendations. Ahsan Shah: “The refiner node is a final step. Think of it as polishing up and editing to synthesize a lot of information gathered prior in the graph and the state, and then compile it together for clear recommendations.”
Traditional AI often focuses on single, isolated tasks. Multi-agent AI, however, involves the collaboration of multiple specialized agents, each with unique capabilities, to tackle complex objectives. This approach mirrors the way human teams work together, allowing for a more comprehensive and nuanced approach to problem-solving.
Instead of replacing finance professionals, multi-agent AI empowers them. By automating routine tasks and providing data-driven insights, these systems free up human experts to focus on strategic thinking, creative problem-solving, and building strong customer relationships.
Building and managing complex multi-agent systems requires advanced engineering and data management capabilities. Ensuring seamless communication between agents, maintaining data security, and establishing clear human oversight are crucial considerations.
Billtrust takes a multi-layered approach to security. Access to sensitive data is strictly controlled, and AI agents operate within carefully defined boundaries. Human oversight is built into the system to ensure responsible decision-making and mitigate potential risks.
Ahsan Shah: “At the tenant level, it’s secured by Snowflake authentication. At the field level, we decide what data to bring in. This isn’t specific to agents; it’s just that agents use tools that use the data. There’s an abstraction between the agent and the data, similar to what we’re already doing with Copilot. So, that’s one guardrail.”
“Another important guardrail is determining what agents can do and how much human oversight is needed. We’re not considering autonomous agents at this point. They won’t adjust policies on the fly; they’ll recommend changes, but won’t have written access. The AI assists you but doesn’t make final decisions.”
As AI technology continues to evolve, multi-agent systems will become even more sophisticated and powerful. We can expect to see increased automation, deeper insights, and more personalized solutions, ultimately leading to a more efficient and strategic approach to AR management.